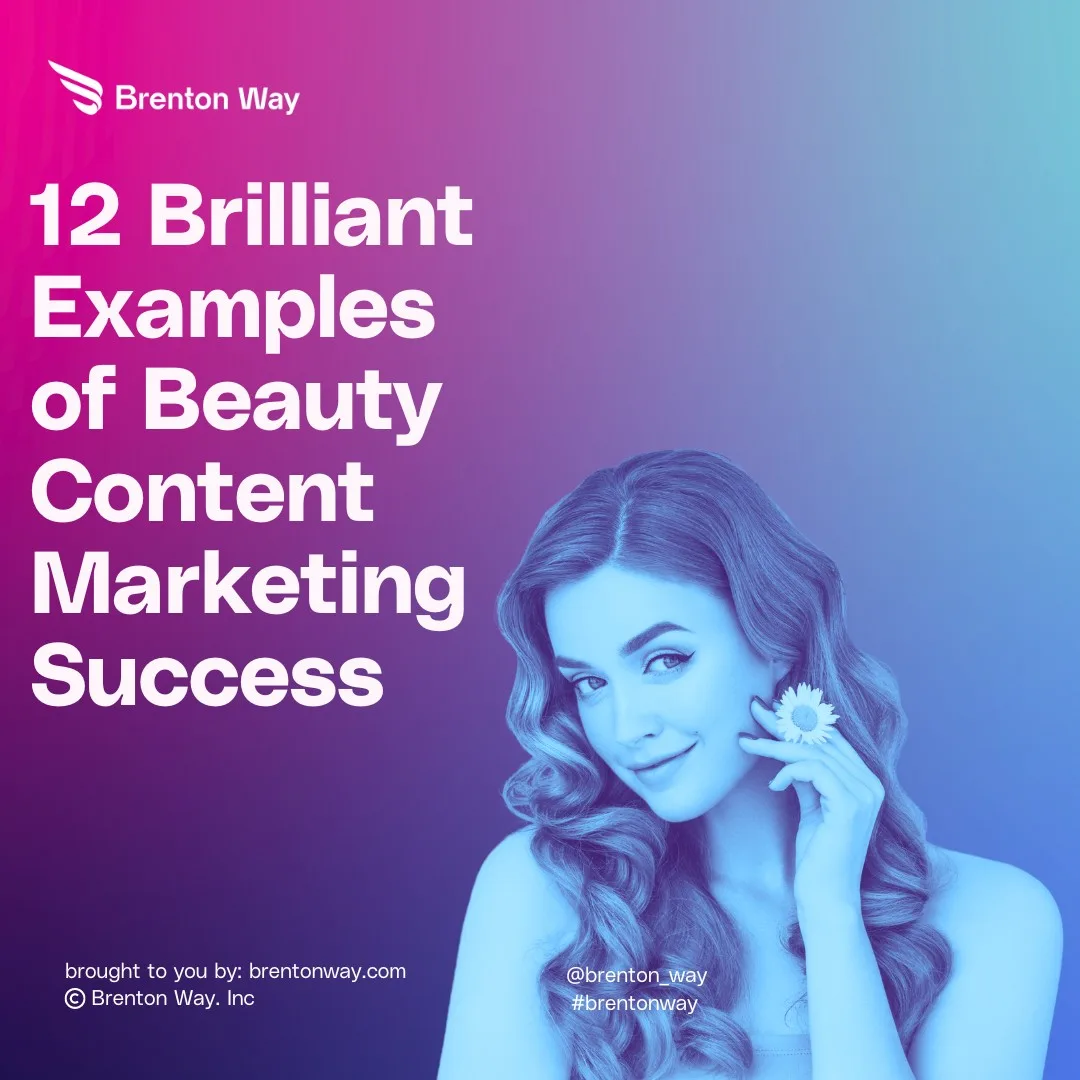
Marketing has evolved beyond catchy slogans and eye-catching visuals. It’s now a science, an art form driven by data. The data-driven approach doesn’t just offer insights; it empowers you to predict, personalize, and perfect your marketing efforts. Companies that use data-driven advertising are six times more likely to be profitable year-over-year. Moreover, personalized email campaigns […]
Marketing has evolved beyond catchy slogans and eye-catching visuals. It’s now a science, an art form driven by data.
The data-driven approach doesn’t just offer insights; it empowers you to predict, personalize, and perfect your marketing efforts.
Companies that use data-driven advertising are six times more likely to be profitable year-over-year.
Moreover, personalized email campaigns based on data deliver six times higher transaction rates. The numbers don’t lie – data-driven marketing delivers results.
Ready to explore our data-driven marketing guide? In this article, we will learn the fundamentals, strategies, and cutting-edge technologies that comprise the world of data-driven marketing.
Data-driven marketing is a strategic methodology that leverages data analysis to guide marketing strategies. It encompasses the collection and thorough examination of data from a variety of sources to gain comprehensive insights into customer behavior, preferences, and emerging trends.
By leveraging these insights, marketers can create more targeted and personalized campaigns. This method allows marketers to allocate resources more efficiently by identifying the most promising audience segments for their products or services.
It also involves measuring marketing campaigns’ return on investment (ROI) to ensure that resources are used effectively.
Data-driven marketing utilizes analytics and predictive modeling to forecast future buyer behavior and make informed decisions about marketing strategies. It emphasizes the importance of data privacy and compliance with relevant regulations while delivering tailored customer experiences.
Also See: Future of Digital Marketing: Predictions And Trends
The world’s first recognized data-driven marketing campaign is often attributed to the American department store chain Montgomery Ward in the late 19th century. In 1872, Aaron Montgomery Ward, the company’s founder, conducted one of the earliest instances of direct mail marketing.
The campaign involved sending a one-page mailer to a list of potential customers, which included farmers and rural residents. The mailer contained a list of items available for purchase, along with their prices. This approach, relying on data-driven decision-making, was revolutionary for its time.
What made this campaign data-driven was Ward’s use of customer data, specifically mailing addresses and purchasing history, to create a targeted and personalized marketing message. He understood the importance of reaching a specific audience with tailored offers, a principle that remains at the core of data-driven marketing today.
Montgomery Ward’s innovative campaign began a new era in marketing, where businesses started leveraging data to understand better and connect with their customers. It set a precedent for the data-driven marketing strategies that would evolve and flourish in the decades and centuries.
Here are the different types of data-driven marketing:
This approach hinges on the examination of past customer actions. Analyzing interactions such as website visits, clicks, purchases, and email engagement helps tailor marketing strategies to align with historical behaviors.
Predictive analytics is a cornerstone of data-driven marketing. Leveraging historical data, it forecasts future customer behavior. By discerning patterns and trends, businesses can proactively create campaigns and strategies to meet evolving customer needs.
Data-driven marketing often involves grouping your audience into distinct categories based on shared attributes or behaviors. This segmentation enables highly targeted marketing campaigns. For example, you might craft different messaging for first-time visitors than loyal customers.
Personalizing content is at the heart of data-driven marketing. By scrutinizing customer data, you can deliver customized content to individual users. This could encompass tailored product recommendations, personalized email subject lines, or website content, enhancing the overall customer experience.
Geolocation data is of particular importance in specific industries as it allows businesses to send location-specific emails or offers to users based on their proximity to physical stores or events.
This strategy is especially relevant for businesses with physical brick-and-mortar locations.offers to users based on their proximity to physical stores or events. This approach is particularly pertinent for businesses with brick-and-mortar locations.
Data-driven marketing spans multiple channels, including email, social media, search engines, and beyond. Data-driven marketers employ data to ensure a unified and omnichannel customer experience across all touchpoints, reinforcing brand identity and elevating customer engagement.
Some marketing strategies pivot on real-time data, where decisions are made instantaneously based on current customer actions. For instance, e-commerce sites utilize real-time data to showcase dynamic product recommendations or personalized offers as customers navigate their platforms.
Data-driven marketing isn’t solely about acquiring new customers; it also nurtures existing ones. Businesses can design loyalty programs and targeted retention campaigns to foster enduring customer relationships by analyzing customer preferences and past purchases.
Here are the various advantages of data-centric marketing:
Data-driven marketing provides a microscope for examining customer behavior. Beyond broad demographics, we gain access to micro-segmentation, revealing customer preferences, purchase patterns, and even the sequence of actions before conversion. This level of granularity allows us to anticipate needs and tailor offerings in unparalleled detail.
By harnessing real-time data, we can implement dynamic pricing models. These strategies involve adjusting prices based on demand, inventory levels, competitor pricing, and customer data. It ensures maximum revenue by charging the right price to customers at the right time.
With predictive analytics, we forecast future behavior and identify potential churn candidates. By proactively addressing the needs of at-risk customers, we can implement retention strategies and prevent customer loss.
Data-driven marketing extends beyond immediate conversions. It focuses on maximizing customer lifetime value (CLV). By analyzing historical data, we can identify high-value customer segments and nurture them with specialized marketing efforts, ultimately increasing CLV.
The rise of voice and visual search demands a data-driven approach. Understanding the nuances of user queries, image recognition, and context enables us to optimize content and ad strategies specifically for these emerging search methods.
Advanced data analytics can delve into sentiment analysis, uncovering customer emotions and opinions from unstructured data sources like social media and reviews. This insight informs messaging strategies, allowing us to resonate emotionally with our audience.
Beyond traditional metrics, cohort analysis examines groups of customers who share a common experience or characteristic. It unveils behavioral trends over time and helps refine marketing strategies for specific customer segments.
Cutting-edge neuroscientific techniques like EEG and eye-tracking provide a window into consumers’ subconscious reactions to marketing stimuli. These insights inform design, messaging, and user experience decisions, optimizing engagement.
Data-driven marketing is increasingly adopting blockchain technology to ensure the security and transparency of customer data. Blockchain verifies data authenticity and builds trust with consumers concerned about data privacy.
Data-driven marketing can empower customers by giving them more control over their data. Implementing consent-based data sharing and transparency practices not only complies with regulations like GDPR but also fosters trust and goodwill.
In essence, data-driven marketing operates by leveraging data to understand the target audience deeply, enabling personalized and optimized marketing efforts that improve customer engagement and business outcomes.
Here are the top challenges associated with data-driven marketing:
Here are the steps to perform data-driven marketing:
Data-driven marketing begins with well-defined objectives.
Imagine you run a subscription-based software company. Your overarching goal is to increase monthly recurring revenue (MRR) by 25% next year. To achieve this, you’ve set specific goals, such as:
A specific goal helps you align your data efforts toward achieving measurable outcomes.
Relevant data can encompass various types, depending on your business.
For instance, if you operate an e-clothing shop, you’d collect data on website visitors, such as their location, browsing history, and purchase behavior.
To collect relevant data, you can:
Tools like Google Analytics can gather this data, offering insights into user demographics and preferences.
Marketing technology tools play a vital role in data-driven marketing.
To choose the right tools, you can:
Audience segmentation involves categorizing your customer base into distinct groups with shared characteristics.
Imagine you run an online clothing store. After analyzing your customer data, you discover that your customers can be grouped into the following segments:
You create tailored email campaigns for each segment featuring products and promotions that align with their preferences.
As a result, you see improved click-through rates and higher sales conversions.
Segmenting your audience empowers you to deliver content that resonates with each group, ultimately driving better marketing outcomes and customer satisfaction.
This segmentation enables you to craft personalized messages and offers for each group.
Using data, you can personalize your marketing materials. Here is the process to follow:
For instance, Netflix uses data on viewer preferences to recommend movies or TV shows, enhancing user engagement.
Suppose you operate an online travel agency. Among your audience segments, you’ve identified “Adventure Seekers” who frequently book adventurous vacations and “Family Travelers” who prefer family-friendly destinations.
For your “Adventure Seekers” segment, you create personalized blog posts and emails featuring thrilling adventure travel packages, hiking tips, and extreme sports recommendations.
Meanwhile, for “Family Travelers,” you craft content highlighting family-friendly resorts, travel itineraries, and kid-friendly activities.
By offering tailored content, you ensure that each segment receives information that aligns with their interests and travel preferences, leading to increased engagement and bookings.
Similarly, e-commerce websites often showcase personalized product recommendations based on a customer’s browsing and purchase history.
A/B testing is a common practice in data-driven marketing.
For example, if you’re an app developer, you might test two versions of an in-app purchase screen to see which design yields higher conversion rates.
This experimentation helps you make data-informed decisions about what works best for your audience.
Key performance indicators are metrics that align with your goals.
Here are the top KPIs to consider for your data-driven marketing campaign:
Metric | Description |
Website Traffic | Measures the number of visitors to your website, providing insights into its visibility and popularity. |
Conversion Rate | Represents the percentage of website visitors who take a desired action, such as making a purchase. |
Bounce Rate | Indicates the percentage of visitors who leave your site after viewing only one page. |
Click-Through Rate (CTR) | Reflects the proportion of clicks on a particular link or ad relative to the total number of times it was displayed. |
Customer Acquisition Cost (CAC) | Measures the cost of acquiring a new customer through marketing efforts. |
Customer Lifetime Value (CLV) | Calculates the anticipated total revenue from a customer over the course of their engagement with your business. |
Churn Rate | Indicates the proportion of customers who discontinue using your product or service within a defined timeframe. |
Return on Investment (ROI) | Assesses the return generated from marketing activities in relation to the total cost of those activities. |
Email Open Rate | Indicates the percentage of recipients who open your email marketing messages. |
Lead-to-Customer Conversion Rate | Calculates the percentage of leads that progress to become paying customers. |
Social Media Engagement | Measures the degree of engagement and activity observed on your social media posts. |
Customer Satisfaction (CSAT) | Gauges customer satisfaction with your product or service through surveys. |
Net Promoter Score (NPS) | Assesses customer loyalty and willingness to recommend your brand. |
Cost per Click (CPC) | Represents the cost paid for each click on online ads. |
Cost per Conversion (CPA) | Reflects the cost of acquiring a customer, calculated as the cost per conversion. |
Average Order Value (AOV) | Measures the average value of each customer order or purchase. |
Click-to-Open Rate (CTOR) | Indicates the percentage of email recipients who both opened the email and clicked on a link within it. |
Marketing Qualified Leads (MQLs) | Represents leads more likely to become customers based on specific criteria. |
Here are some of the leading examples of data-driven marketing for your inspiration: